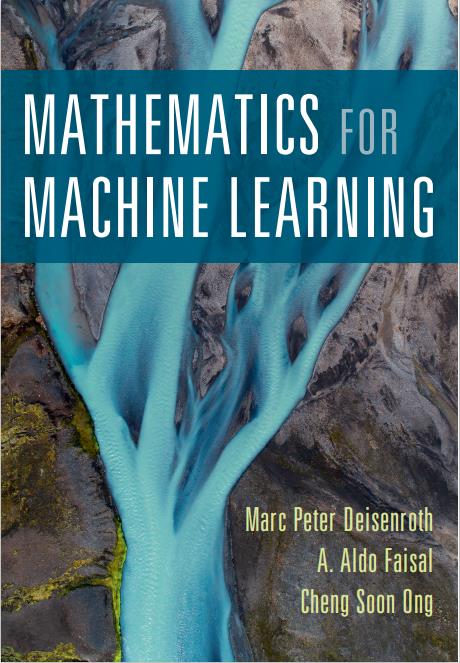
内容简介
This self-contained textbook introduces all the relevant mathematical concepts needed to understand and use machine learning methods, with a minimum of prerequisites. Topics include linear algebra, analytic geometry, matrix decompositions, vector calculus, optimization, probability and statistics.
The fundamental mathematical tools needed to understand machine learning include linear algebra, analytic geometry, matrix decompositions, vector calculus, optimization, probability and statistics. These topics are traditionally taught in disparate courses, making it hard for data science or computer science students, or professionals, to efficiently learn the mathematics. This self-contained textbook bridges the gap between mathematical and machine learning texts, introducing the mathematical concepts with a minimum of prerequisites. It uses these concepts to derive four central machine learning methods: linear regression, principal component analysis, Gaussian mixture models and support vector machines. For students and others with a mathematical background, these derivations provide a starting point to machine learning texts. For those learning the mathematics for the first time, the methods help build intuition and practical experience with applying mathematical concepts. Every chapter includes worked examples and exercises to test understanding. Programming tutorials are offered on the book’s web site.
获取:
Mathematics For Machine Learning by Marc Peter Deisenroth, A. Aldo Faisal, Cheng Soon Ong.pdf
下载说明:
在下载页面点击“普通下载”即可,可能会弹出第三方广告页面,请忽略。 详细下载教程请点这里:本站下载教程
电子书格式转换及格式科普请点这里:电子书格式